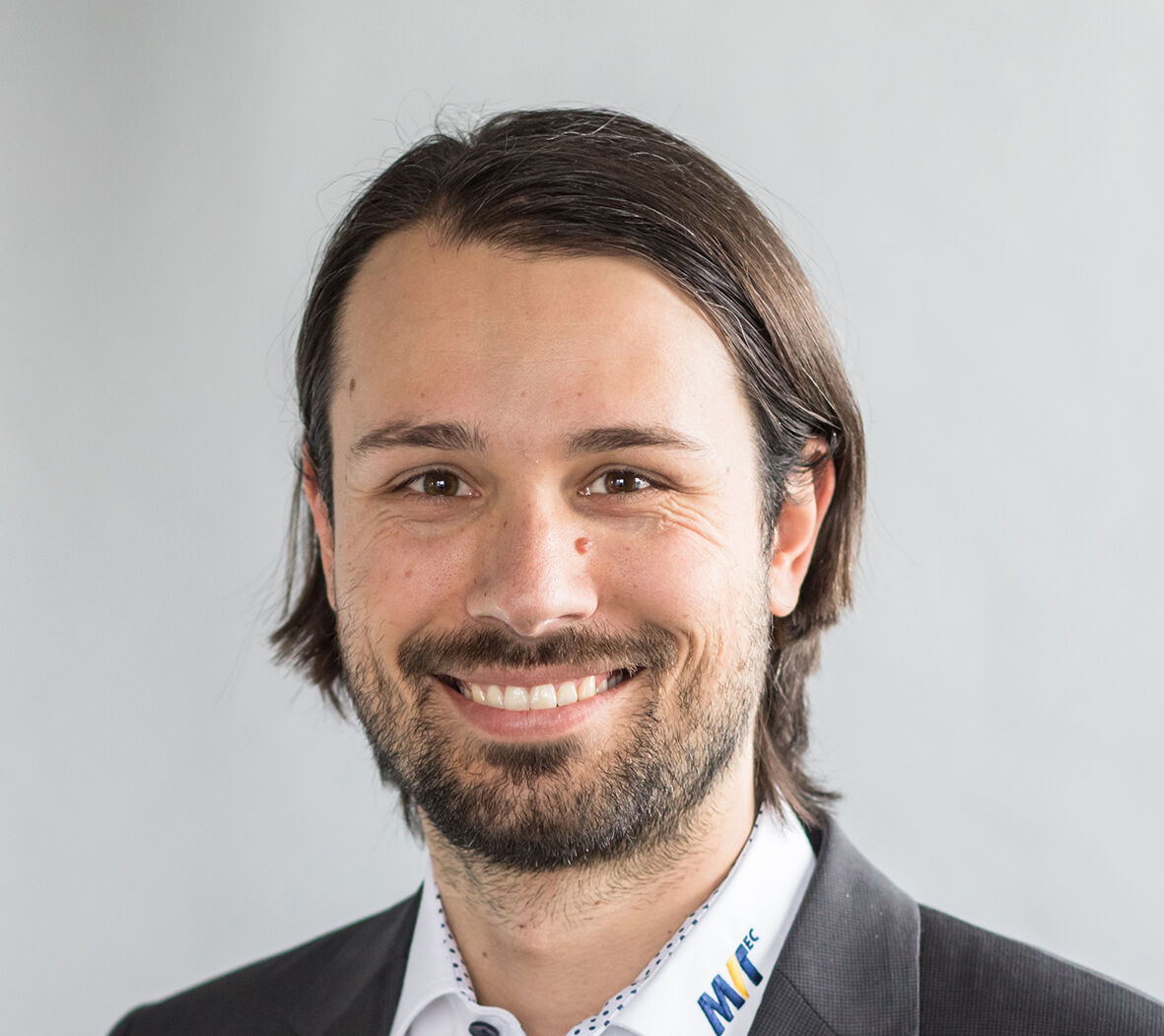
Everyone is talking about AI Vision: Are AI systems already being used?
Peter Droege (Maddox AI): We had a couple of surveys around that topic. 80% of the respondent said that they still operate with manual quality control. 70% said that they believe that AI-based inspection systems are ready for regular production. At the same time only 17% of that persons already use AI systems, so there is a clear gap between understanding that AI can be something that is helpful and actually implementing it. The reasons for that gap are different. One is the costs. Then AI is a new technology and there are a lot of players on the market. So it is pretty hard to understand who is the leading player and who is save to operate with. Is it a startup or better a bigger company? The survey showed that 90% of the time is being spend not for the algorithm part but at the data quality part. Is an error that has been marked by one expert also marked by another expert? Most of the AI systems don’t address data quality with a high enough urgency.
Florian Güldner (ARC Advisory Group): I remember an ARC workshop where someone from Dow Chemicals said it’s fine that all the challenges are not AI challenges, it’s organization, it’s data, it’s people… not saying that there are no technological challenges, but the big challenges which can make or break a project are not AI and technology. AI is already being used. More and more people have company wide, enterprise wide or even (inter)plant wide programs for AI to push that technology and they try to scale it as much as possible.
Daniel Routschka (IDS): We need to differentiate because there are different applications. If we look at embedded systems it is already in serious production. In simple applications where in most cases vision sensors are added by an AI component, AI is one tool among many. If you do classifications, simple object detection, Deep Learning OCR, AI is already being used with good results and visible on the shopfloor level. In the higher price segment these applications are often very customer specific and more time consuming and therefore usually linked to non-disclosure agreements. At one of the presentations before DeGould showed that their car inspection tunnel with AI is already being used in eight out of ten major OEMs. In most cases, the companies are just fixed and won´t talk about it. Huge enterprises have AI departments to bring AI into a brighter level in huge applications. AI is already in serious productions but it can be more.
Christian Eckstein (MVTec): Like any new technology, AI has to prove for each application that it is better than the alternative, and this is not necessarily the case. The project costs in total are sometimes more expensive, and deep learning requires a lot of performance, not only in terms of data, but also in terms of processing performance, because sometimes we are talking about cycle times of only a few milliseconds. In production, everything is constantly changing. So you think that your system is running but then something changes. With traditional machine vision you can react just by changing some settings or parameters. With AI you have to retrain and modify your data and go back and restart the entire process.
What is the main problem for users starting with AI?
Droege: It depends on the application. Some cases have a clear benefit and are easy-to-use. But look at a more complex inspection with 20 different classes and probably some of these awesome marketing promises that say everything is OK with fifty samples. That will not be the case. The real difficulty lies in finding a consensus between what people want and what quality experts actually consider to be an error is. If you give the same hundred parts to two different experts and look at the consistence how many of these parts are rated differently between these two experts, the companies‘ expectation is 15%. But the reality that we see in our project is closer to 30%. Because we now digitized this problem, we can solved it. But then it all comes back to the data issue. What we need is some kind of data debugging tool to show where consensus failed and build consensus in terms of data quality.
Eckstein: The problem is not the complexity of the system itself, because conventional machine vision can be just as complex. With AI, the interaction is quite simple: you label data, you have a good data set, train it, and then it works or it doesn´t. I don’t think the system itself is too complex, it´s the process to reach a good model which is complex.
Routschka: From the sales point of view, it’s important to limit the customer’s expectations, somewhat, because in most cases it starts with this one bug thats’s been there for years, and if you can solve this problem with AI, it doesn´t matter in the end, because the customer wants to see more details, solve more defects and wants a whole solution. The expectations may grow within the project and it has to be clear at the beginning what are the expectations and what is the level we need to achieve.
Güldner: That is exactly what happens. Your start with a single problem: ‚I want a solution and AI could be the right technology‘ and then you involve more people in your project and then the scope goes out of proportion and in the end, you pitch it to the management and say: ‚AI solves all our problems‘ and then of course you fail. You have to speak over the application and expectations right at the beginning.
Is AI Vision lowering the barrier for the user, or is it rather about solving unconstrained applications?
Droege: It can be both. There are certain applications where the variability of the real world is so wide that it will be very hard to automate for a classic vision system. There are cases where AI will be beneficial and helps to solve certain tasks that we were not able to solve before. In terms of lowering barriers I would say ‚yes‘, because in many cases you do no longer need a vision or coding expert to create a system, but there are different tasks and complexities that arise. We already talked about the data quality issue. Looking at the data is just a different approach, but it’s not very common right now. So you have to educate the people. More people can use AI systems if they understand the way how to interact with an assistance through image annotations.
Routschka: The number of image based inspections are continuously increasing and you won´t have so many vision experts in the future especially in Germany. This should be the main goal to bring AI applications into the production.
Güldner: You need to serve these mega trends and one of them is the shortage of workers and another is of course sustainability. So AI will penetrate more applications to replace workers that we will not have in the future. This is not due the prices, it’s because of the lack of workers.
How to compare AI performance and how to select the best algorithm for my application?
Eckstein: You really have to define the strategic value of AI for your company. Is it a core element of your strategy where you want to add value yourself by offering AI, than you have to do as much as possible by yourself. If you think it is strategic, but just part of your portfolio and not your core product or competency, then you should look for a partner that you can work with over years to improve your products. If you just look for a very quick solution for one machine or application, then you can do this with an in-house AI expert, an open source tool, or an integrator.
Routschka: There is no such thing as the best universal solution. What we must not forget is customer satisfaction, and this depends not only on hard facts, but also on customization options and feel good factors… How good is the best performance if I can’t find my way around in the system because the setup is to complex? Customers often relay on long partnerships with an integrator because there is something beyond the hard facts like trust. That is something which AI needs to build up as well.
Droege: The issue of trust is important, and obviously the overview of AI companies‘ competitors is so scattered. So it’s pretty hard to see through the smoke. We even allow our customers to test the entire AI system for free in the beginning. We define what the system needs to do, how accurate it needs to be and how fast. Then we actually perform against these KPI´s (Key Performance Indicators) and if we don’t achieve them the customer doesn´t pay anything. In this way, we create trust, because if we did this with all customers and never got an order, this business model would be very short term.
What role does synthetic images play in the future?
Eckstein: Synthetic data plays a big role. What you can do is increase data size and cover edge cases. To be honest, I had the high point of my personal hype cycle with synthetic images two years ago. Then I was really disappointed. Unfortunately synthetic images look real to a human, but they are not real to the AI. The features are too different to the actual real life examples. For defect detection there is a very limited use and it can help for unsupervised methods where you don’t have any defects at all like in anomaly detection. If you just want some images to test a model you can create some synthetic defects. But it can be very valuable when it comes to 3D and you had a CAD model and generate images from that. Then, you can do 60 positions of one part because real 3D images are very expensive for the training data set and usually have a low quality especially with cheaper 3D sensors. For a pick&place application, synthetic images are a good solution.
Güldner: Synthetic imagines make tremendous progress thanks to AI. At the latest photoshop version you can simply tell by voice ‚pigs in the garden‘ and the image is there. If you want to make a check if AI is working or not, separate the teams and then use different approaches that can actually get rid of this circle reference where you create images and defects and than test if you can detect them.
Droege: Every AI company uses synthetic data generation to some extent, but for defect creation I don’t think, it’s the magic bullet. If you have a generative model, which can create all kinds of defects in all it’s variability you have to solve a task. But you need a model to solve the task to generate training data, and you reuse that task again which by definition is not 100% sensible.
Eckstein: Especially if you use generative models. The theory would be that a model generated from defects would add information to your model.
Droege: We have all seen the hype about large language models, but when you look at large vision models there is not much there. Mostly these models have been tested on internet data and this is very different. There are no open source for industry image data sets with the ideal large variability of different defects. This would help tremendously not only for preparing purposes. You can bring down the number of actual samples that you need and so you can work a similar problem from a different angle.
What is about the scalability of AI systems: Is Copy&Paste possible?
Droege: In some case, it is close to it. We have several systems where we scale them up to several lines, and there are these small differences in rotations of position or lighting. It´s not Copy&Paste, but it’s 95% the same, so the extra work is significantly reduced. Then we have this benefit that a rare error in Mexico, can also teach to a similar system that is standing in Germany, for example.
Eckstein: In theory Copy&Paste should be possible, if the new conditions and images are represented by the data set that the model was trained on. Often, this is not the case, because conditions like lighting change or the background color of a conveyor belt is different. The way we want to solve this problem is with Continual Learning. You train a basic model and then use it with a few images on the production side. You just train on the CPU with an additional hundred images, which only takes a minute.
Is Continual Learning with some few images really deployed in production?
Eckstein: The general problem in retraining a model, with Continual Learning, is that you put only a few images in a data set and hope that it’s better than before. However, those methods that we had in the past were like humans. Humans greatly overvalue current information over old information. What these models suffered from was termed catastrophic forgetting, meaning although they could classify the new images, they couldn’t classify the old images anymore.
So if you have a classification model with three classes and you want to add a fourth class with a new defect type, some companies in the past added a branch only to one class, which ended up not being a good solution.
Routschka: On an embedded system, Copy&Paste depends on the case and how stable it is. If it’s an application that’s going to be distributed to different production facilities, then the setup is quite different from a one-off application. On the other hand, I’ve tried it several times with our NXT system with applications from different people and in different locations, and it works. It depends what you want to detect. If you’re looking for the micro scratches or trying to detect the apple / banana stuff or something else, those are already pretty robust applications. Even for industrial applications like solder inspection, there are pretty good basic models with a good data base.
Güldner: Many models tend to drift over time. So even if you do Plug&Play you have to re-adjust them after a while. Of course it depends on the setup and if you can do everything remotely or how you implement and then retrained it. Furthermore, you have to look at the future and the very flexible production layouts. This means that the repeatability of the plants looks less and less like the other. These decreased complexity on the plant floor makes it complicated just to reuse the models. The target should be – and we will get there – that more than 99% will be reusable. A good strategy to increase repeatability and scalability is a layered model. So we can have a core technology that identified defects, and on top of that a layer that is suitable for electronics and another layer for automotive industry. You should be able to enlarge parts of your model, but you don’t need to enlarge the entire model.
Rotschka: There are so many factors that influence this issue, for example, how many parts suppliers do you have in production? It happens all the time that you go into production because the AI system is not working and you ask: ‚Did you change anything? No no, everything is fine‘ and then you figure out that the customer has a new supplier for the plastic parts. That is something they have to be aware of and most of these factors are also faced by common computer vision that we had in the past as well. This can´t be an excuse, but it need to be a continuous improvement and that’s what people are also expecting from AI usage. We are developing over a certain period and getting better every time.
Will autonomous vision systems be possible in the future thanks to AI?
Eckstein: Where do we have the fastest breakthroughs with AI? We had them where we had big data sets and clearly defined problems. With ChatGPT we had big data as text. The more you can generalize the problem, the more people have exactly the same problem, the more you can move towards an autonomous vision system. For very generalized applications like surveillance or Deep OCR, you can have more and more autonomous systems that analyse this applications in general. For a very specific problem like spot welding, which has a defect underneath and somehow the AI should see that through the weld, that’s a very specific problem and there will be not a generalized AI solution.
Routschka: I think for simple cases like code reading or OCR, there are already these kinds of self-learning AI tools where you simply illuminate with different light factors and then figure out which the best image to start with. In more complex cases like quality inspection with different perspectives, it will take at least a few more years until we get there.
Güldner: There will be applications where we can actually do that in the near future. The whole AI or vision complexity will be taken away from the user – because of shortage of workers – and it will work as if it’s autonomous, but human AI experts will be in the loop all over the world, teaching, training and optimizing the model in the background.
n Florian Güldner, Managing Director, ARC Advisory Group
n Daniel Routschka, Sales Manager AI, IDS
n Peter Droege, CEO, Maddox AI
n Christian Eckstein, Business Developer, MVTec Software
Moderation: Dr.-Ing. Peter Ebert, Editor in Chief, inVISION